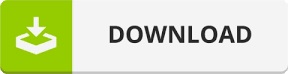
For the incongruity module, we create positive (incongruous) examples by pairing product names with questions extracted from other products’ pages. Those scores are concatenated with ordinary word embeddings - vector representations that capture semantic information about the inputs - before passing to a classifier, which makes the ultimate decision about whether the question is comic.īefore training the network as a whole, we pretrain the incongruity and subjectivity modules on automatically labeled data. The architecture of our humor detection network, with pretrained modules for recognizing incongruity and subjectivity. Both the title and the question pass to an incongruity detection module, which scores them according to incongruity, and the question passes to a subjectivity module, which scores it according to subjectivity. The inputs to our system are a question extracted from an Amazon product page and the associated product title.
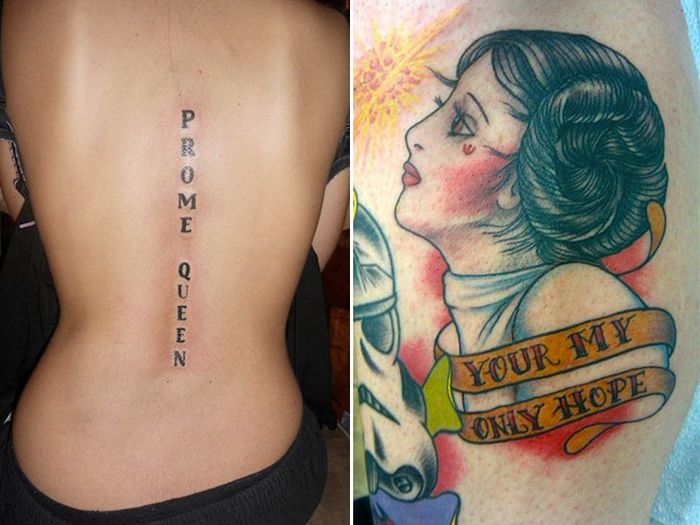
For instance, one question asked about the Amazon Echo Show was the comic question “Will this help me find the meaning of life?”, which has a more subjective tone than the question “Can it connect to music speakers?” The other insight is that humor often has a subjective tone, an indication of the speaker’s sentiment or emotional state. For instance, the question “Does this make espresso?” might be reasonable when applied to a high-end coffee machine, but applied to a Swiss Army knife, it’s probably a joke. One is that humor is often the result of incongruity - a mismatch between two conceptions of a topic. Our system leverages two insights from humor theory. In experiments, we compared our system to four baselines, and it reduced the error rate of the best-performing of them by 5.4% and 18.3% on two different data sets. In a paper presented (virtually) at this year’s SIGIR, the Association for Computing Machinery’s annual conference on information retrieval, my colleagues - Yftah Ziser and Elad Kravi - and I described a new approach to humor detection in product question answering.
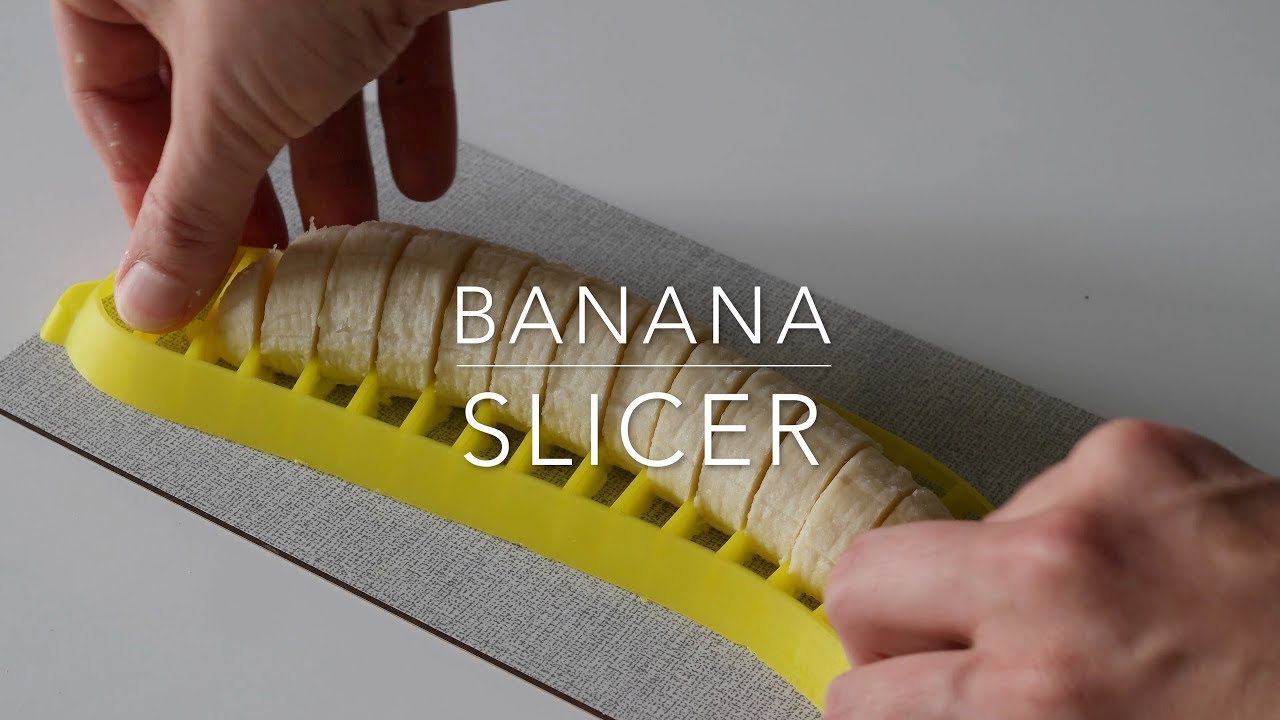

But for customers in a hurry to extract essential information about products - and for automated systems that use question-and-answer data to improve Amazon’s recommendation engine - it would be useful to be able to distinguish comic from serious questions. Providing new opportunities for creative self-expression is one of the delights of building an online community like Amazon’s.
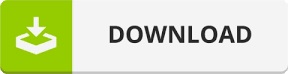